An integrality gap for the planted clique problem
The Planted Clique problem (sometimes referred to as the hidden clique problem) is a central question in average-case complexity - where one is interested in the computational complexity of solving typical (as against worst-case) instances. The problem is rooted in the 1976 work of Karp (Karp 1976) that asked to find a maximum clique (i.e., set of vertices that are all neighbors of one another) in a Erdös-Rényi random graph \(G(n,\frac{1}{2})\) (where every edge is included independently in the graph with probability \(\frac{1}{2}\) independently).In such a graph it can be shown that with high probability the maximum clique will be size at least \((2-o(1))\log n\) but the simple greedy algorithm only recovers a \(\log n\) sized clique and it is a longstanding open problem to recover a clique of size \((1+\epsilon)\log n\) for every constant \(\epsilon>0\). Jerrum (Jerrum 1992) and Kucera (Kucera 1995) defined Planted Clique as a relaxation of this problem: for some \(\omega \in \{1,\ldots,n \}\), find an \(\omega\) clique added to an Erdös-Rényi random graph \(G \sim G(n,\frac{1}{2})\). That is, we choose \(G\) as a random graph from \(G(n,\tfrac{1}{2})\), choose \(S\) to be a random \(\omega\)-sized subset of \([n]\) and then add to \(G\) all the edges between pairs of vertices in \(S\). As the exercise below shows, the problem can be solved by brute force search in quasi-polynomial time as long as \(\omega \gg \log{(n)}\).
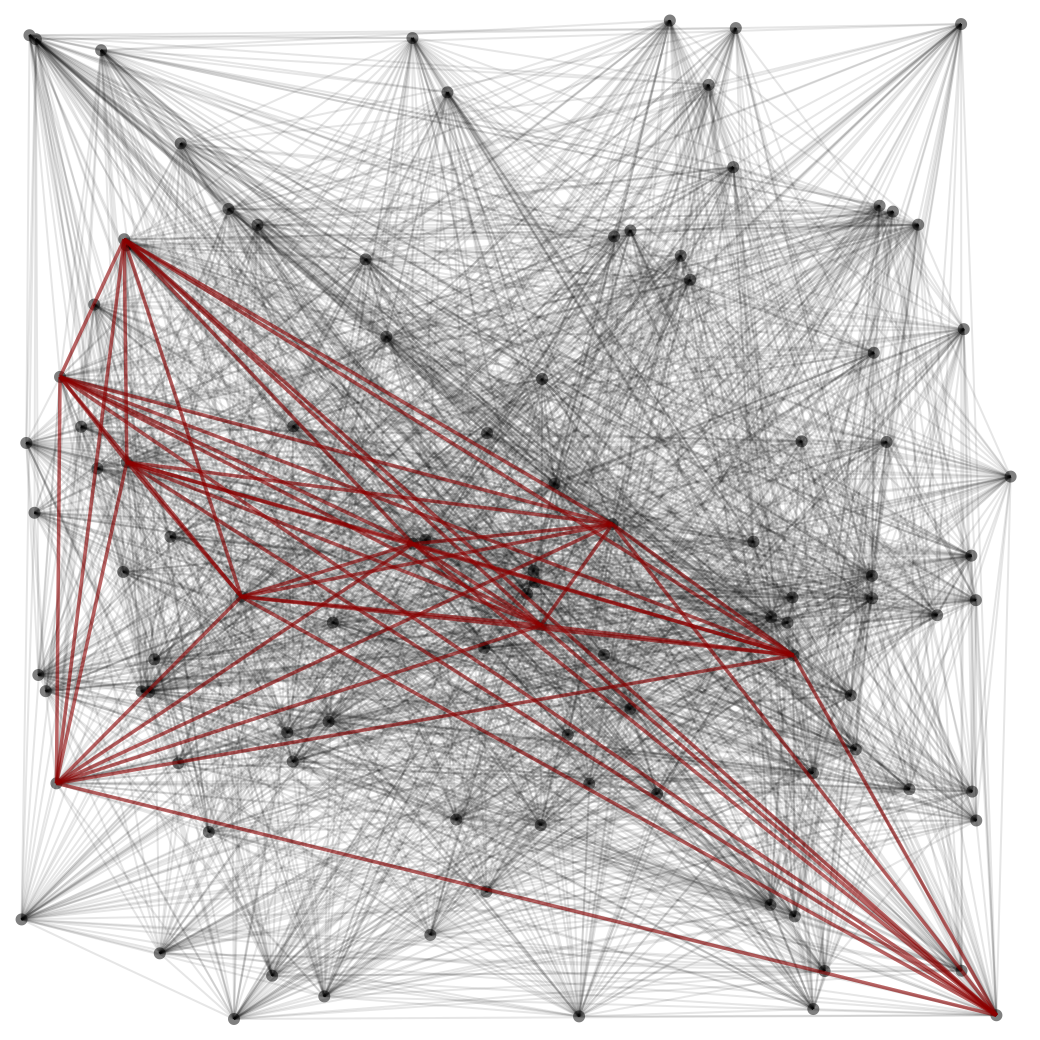
Show that the maximum clique in a random graph is of size \(c \log{(n)}\) with probability at least \(0.99\). How small can \(c\) be? Use this observation to obtain a \(n^{O(\log{(n)})}\) time algorithm to find planted cliques of any size \(\omega \gg \log{(n)}.\)
In recent years, planted clique and related problems have found applications to important questions in a variety of areas including community detection (Hajek, Wu, and Xu 2015), finding signals in molecular biology (Pevzner, Sze, and others 2000), discovering motifs in biological networks (Milo et al. 2002; Javadi and Montanari 2015), computing Nash equilibrium (Hazan and Krauthgamer 2009; Austrin, Braverman, and Chlamtac 2013), property testing (N. Alon et al. 2007), sparse principal component analysis (Berthet and Rigollet 2013), compressed sensing (Koiran and Zouzias 2014), cryptography (Juels and Peinado 1998; Applebaum, Barak, and Wigderson 2010) and even mathematical finance (Arora et al. 2010).
One would expect finding added cliques to become easier with increasing \(\omega\). Indeed, when \(\omega > C \sqrt{n \log{(n)}}\) for some large enough constant \(C\), one can recover the vertices of the added clique by simply collecting the highest degree vertices. A more clever algorithm uses the spectrum of the adjacency matrix of the graph to find added cliques of size \(\Theta(\sqrt{n})\). The following exercise illustrates the idea for the distinguishingThe different variants of the planted clique problem: Like other average-case problems in \(\mathbf{NP}\), the planted clique problem has three natural variants of search, refutation, and decision. The search variant is the task of recovering the clique from a graph in which it was planted. The refutation variant is the task of certifying that a random graph in \(G(n,\frac{1}{2})\) does not have a clique of size \(\omega\). The decision problem is to distinguish between a random graph from \(G(n,\frac{1}{2})\) and a graph in which an \(\omega\)-sized clique has been planted. The decision variant can be reduced to either the search or the refutation variant, but a reduction between the latter two variants is not known. variant of the problem.
Let \(B(G)\) be the \(\{\pm1\}\)-adjacency matrix for graph \(G\), i. e., \(B(i,j) = +1\) if \(\{i,j\}\) is an edge in \(G\) and \(-1\) otherwise.
- Show that the maximum eigenvalue of \(B(G)\) for a random graph \(G\) is at most \(\Theta(\sqrt{n})\) with probability at least \(0.99\).
- Show that the maximum eigenvalue of \(B(G)\) for \(G\), a random graph with an added clique of size \(\omega\) is \(\Omega(\omega).\)
- Conclude that when \(\omega > C\sqrt{n}\) for some large enough constant \(C\), the maximum eigenvalue of \(B(G)\) can be used to detect whether a random graph has an added planted clique of size \(\omega\).
- Can you modify this procedure to give a polynomial time algorithm that can distinguish between \(G\) which is random and \(G\) that has an added clique size \(\omega > \epsilon \sqrt{n}\) for any constant \(\epsilon >0\)?
We note that an even simpler distinguishing algorithm can be obtained by simply comparing the total number of edges, as when \(\omega\gg \sqrt{n}\) the expected number \(\tfrac{1}{2}\binom{\omega}{2}\) of edges we add will be larger than the standard deviation \(\sqrt{\binom{n}{2}}\) of the total number of edges. However, the spectral based algorithm can be generalized better to the search problem, and is also a more insightful starting point to the sos discussion.
Despite being intensely studied, state-of-the-art polynomial time algorithm for the problem is essentially the one based on the exercise above and works for \(\omega = \epsilon \sqrt{n}\), for any constant \(\epsilon>0\) (N. Alon, Krivelevich, and Sudakov 1998). On the other hand, it is unlikely that lower bounds for planted clique can be derived from conjectured separations in worst-case complexity classes such as \(\mathbf{P}\neq \textbf{NP}\), precisely because it is an average-case problem (Feigenbaum and Fortnow 1991; Bogdanov and Trevisan 2003). As a result, our best evidence for the difficulty of the problem comes from showing limitations on powerful classes of algorithms. In particular, since many of the algorithmic approaches for this and related problems involve spectral techniques and convex programs, limitations for these types of algorithms are especially interesting. One such negative result was shown by Feige and Krauthgamer (2003) who proved that a weaker hierarchy than sos- namely, the \(n^{O(d)}\)-time degree \(d\) Lovàsz-Schrijver semidefinite programming hierarchy (\(LS_+\) in short) can only recover the added clique if its size is at least \(\sqrt{n/2^d}\).Formally such results apply to the incomparable refutation problem, which is the task of certifying that there is no \(\omega\)-sized clique in a random \(G(n,\frac{1}{2})\) graph. However, our current knowledge is consistent with all three variants having the same computational complexity.
In this chapter, we will find out if the SoS algorithm can help in detecting planted cliques of size \(o(\sqrt{n})\). While the answer will be somewhat disappointingly negative, our investigation will illuminate certain new aspects of the SoS algorithm. This would naturally lead to the idea of looking at SoS and similar algorithms (i.e. those with an associated proof system) as implementing a computationally bounded version of classical Bayesian reasoning and allow us to interpret ``state’’ of an algorithm even when it fails to solve a given computational problem. Such an interpretation will also illustrate how the SoS algorithm is more powerful than other semidefinite programming based methods such as the \(LS_+\) algorithm.
The integrality gap we prove in this section will show that the SoS algorithm of degree \(d\) ``thinks’’ that there is a \(> n^{1/2 - c(d/\log{(n)})^{1/2}}\)-size clique in a random graph with high probability. For any \(d = o(\log{(n)})\), the above bound on \(\omega\) equals \(\sqrt{n}\) up to \(n^{o(1)}\) factors. As in the case of Grigoriev’s theorem for Max \(3\)XOR problem, this amounts to constructing a degree \(d\) pseudodistribution supported on \(\approx \omega\)-cliques in a random graph \(G\). We encode a clique \(S \subseteq [n]\) as its characteristic vector \(x\in\bits^n\), where the condition of being a clique corresponds to the constraint that \(x_ix_j=0\) for every non edge \(\{i,j\}\). Formally, we will show the following result of Barak et al. (2016):
Let \(d = d(n)\) be some function. Then, there is an absolute constant \(c\) such that for \(\omega = n^{\frac{1}{2} - c (d/\log{(n)})^{1/2}}\) and for large enough \(n\), with probability at least \(1-1/n\) over \(G \sim G(n,\frac{1}{2})\), there is a degree \(d\) pseudodistribution \(\mu\) over \(\bits^n\) consistent with the constraints \(\{x_ix_j = 0\}\) for every \(i \not \sim j\) in \(G\) such that \(\pE \sum_{i=1}^n x_i \geq \omega\).
Planted Clique Versus Max \(3\) XOR
How should we construct a pseudodistribution that pretends that there’s a \(\approx \omega\)-clique in a random graph? We could look back on the proof of Grigoriev’s theorem to draw some inspiration. The construction of the pseudodistribution in case of Max \(3\)XOR appears natural in retrospect - in a sense, we find out the “hard” constraints imposed by the input instance by performing a bounded width derivation and choose the target pseudodistribution to be as random as possible after forcing it to satisfy the hard constraints. In the Bayesian context we can justify this via Jaynes’s maximum entropy principle. That is, we start with the most uninformative prior of the uniform distribution over \(\bits^n\), and add the minimum constraints that we have observed from the instance. (Of course if we truly derived all the logical consequences of the instance then we would see that there is no satisfying assignment, but we restrict ourselves to bounded width derivation.)
This is possible in Max \(3\)XOR because the effect of one subset of variables on another is either extremely strong (if they are “nearby” in the bipartite instance graph \(G\)) or essentially zero. For example, if we have a 3XOR constraint \(x_1 \oplus x_2 \oplus x_3 = 1\) then knowing \(x_1\) and \(x_2\) completely determines the value of \(x_3\). However, if there was no short chain of constraints linking \(x_1,x_2\) and \(x_3\) then we can think of \(x_3\) as being independent of \(x_1,x_2\). Indeed, in Grigoriev’s pseudo-distribution, for every subset \(S\subseteq [n]\), the expectation of \(\chi_S(x) = \prod_{i\in S}(1-2x_i)\) was either equal to \(0\) or in \(\{ pm 1\}\). If this was an actual distribution over \(x\in\bits^n\) then since \(\chi_S\) is \(\{\pm 1\}\) valued it means that either \(\chi_S(x)\) is completely uniform or it is fixed to one particular value.This intuition of correlations quickly “dying out” as variables get further away from one another is widely used also outside of sos, and in particular is key for many analysis of algorithms such as belief propagation for constraint satisfaction problems. Such correlation decay can be shown formally for underconstrained random 3SAT or 3XOR where there is a large number of satisfying assignments, and so there exists an actual probability distribution over the assignments. In our “overconstrained” regime there will exist only one (in the planted setting) or no solution (in the random setting) and so we can only talk about “Bayesian” pseudo-probabilities.
In contrast to the strong local effects we see for constraint satisfaction problems, it turns out that for the Planted Clique problem, every variable has a weak, but global effect on all the other variables. Consider a random graph \(G\) in which a clique \(S\) of size \(\omega\) has been planted. If someone tells us that vertex \(17\) is not in \(S\), this new information makes it slightly less likely that \(17\)’s neighbors are in \(S\) and slightly more likely that \(17\)’s non-neighbors are in \(S\). So, this information has a weak global effect.In contrast, if someone tells us that \(17\) is in \(S\) then that has a strong effect on \(17\)’s non-neighbors, and weak effect on its neighbors. Note however that the planted clique problem is only non-trivial when \(\omega\ll n\) and so every vertex is much more likely to be outside the clique than the other way around. This is in contrast to the strong local effects that we see for constraint satisfaction problems such as random 3XOR. This difference between the random Max \(3\)XOR (and other constraint satisfaction problems or CSPs) and the planted clique problems means that some subtleties that can be ignored in setting of random CSPs need to be tackled head-on when dealing with planted clique.
Computationallly Bounded Bayesian Estimates for Planted Clique
Let \(G(n,1/2,\omega)\) be the distribution over pairs \((G,x)\) of an \(n\)-vertex graphs \(G\) and a vector \(x\in\R^n\) which is obtained by sampling a random graph in \(G(n,1/2)\), planting an \(\omega\)-sized clique in it, and letting \(G\) be the resulting graph and \(x\) the \(0/1\) characteristic vector of the planted clique. Let \(f:\{0,1\}^{\binom{n}{2}}\times \R^n \rightarrow \R\) be some function that maps a graph \(G\) and a vector \(x\) into some real number \(f(G,x)\) which we’ll write as \(f_G(x)\). Now imagine two parties, Alice and Bob (where Bob stands for “Bayesian”) that play the following game: Alice samples \((G,x)\) from the distribution \(G(n,1/2,\omega)\) and sends \(G\) to Bob, who wants to output the expected value of \(f_G(x)\). We denote this value by \(\pE_G f_G\).
If we have no computational constraints then it is clear that Bob will simply output \(\E_{x|G} f_G(x)\), i. e., the expected value of \(f_G(x)\) where \(x\) is chosen according to the conditional distribution on \(x\) given the graph \(G\).It might seem a bit disconcerting to talk of an expectation when with high probability, the associated distribution has support of size one: the added planted clique. However, we will soon move on to the setting of pseudodistributions where we’ll be able to pretend as if there is not one but many large cliques in a random graph. In particular, the value \(\pE_G f_G\) will be calibrated in the sense that \[ \E_{G \in_R G(n,1/2,\omega)} \pE_G f_G = \E_{(G,x) \in_R G(n,1/2,\omega)} f_G(x) \label{eq:calibration} \]
Now if Bob is computationally bounded, then he will not necessarily be able to compute the value of \(\E_{x|G} f_G(x)\) even for a simple function such as \(f_G(x)=x_{17}\). Indeed, as discussed before, since with high probability the clique \(x\) is uniquely determined by \(G\), \(\E_{x|G} x_{17}\) will simply equal \(1\) if vertex \(17\) is in the clique and equal \(0\) otherwise and hence accurately computing \(\E_{x|G} x_i\) for all \(i\) corresponds to being able to recover the clique. However, note that we don’t need to compute the true conditional expectation to obtain a calibrated estimate! Simplying outputting \(\pE x_{17} = \omega/n\) will satisfy Equation \eqref{eq:calibration}.
However, calibration does require us to get certain correlations right.
Consider the function \(f_G(x) = (vdeg_G(17)-n/2)(x_{17}-\omega/n)\),
where \(vdeg_G(i)\) corresponds to the degree of the vertex \(i\) in the
graph \(G\). This function captures the covariance of the degree of the
vertex with the event that it is in the clique.
Naturally higher degree vertices are somewhat more likely to be in the
clique, but satisfying \eqref{eq:calibration} with respect to this
\(f\) means that we must get this correlation right. This makes sense,
clearly Bob can compute a priori this correlation and so if his
estimates do not achieve it then they are clearly “leaving some
information on the table”. In particular this means that if we want to
satisfy the calibration condition with respect to this function \(f\),
then the value \(\pE_G x_i\) should not be fixed to \(\omega/n\) but rather
be correlated with the degree of \(x_i\).
Show that if a pseudo-expectation operator \(G \mapsto \pE_G\) is calibrated with respect to all functions \(f\from\bits^{\binom{n}{2}}\times\bits^n\to\R\) that have the form \(f_G(x) = g(G)x_i\) where \(g\from\bits^{\binom{n}{2}}\to\bits\) is an arbitrary function then it must be that \(\pE_G x_i = \E_{x|G} x_i\). In particular this means that with high probability over \(G\) it holds that \(\pE_G x_i \in \{0,1\}\) for every \(i\).
Pseudocalibration
The above discussion suggests that the pseudodistribution we construct shouldn’t distinguish between a graph \(G\) drawn from \(G(n, \frac{1}{2})\) and a random \(G\) from \(G(n,\frac{1}{2},\omega)\). We can capture this by the notion of being “pseudocalibrated for a function \(f\)” as follows:
A degree \(d\) pseudo expectation map is a function that takes a graph \(G\) into a degree \(d\) pseudo-expectation operator \(\pE_G\) (or equivalently, a degree \(d\) pseudo-distribution \(\mu_G\)).
Let \(f\from\bits^{\binom{n}{2}}\times \bits^n\to\R\). A degree \(d\) pseudoexpectation map \(\pE_G\) is pseudocalibrated with respect to \(f\) if it satisfies: \[ \E_{G \in_R G(n,1/2)} \pE_G f_G = \E_{(G,x) \in_R G(n,1/2,\omega)} f_G(x), \label{eq:pseudo-calibration} \]
Note that \eqref{eq:pseudo-calibration} does not make sense for the estimates of a truly Bayesian (i.e., computationally unbounded) Bob, since almost all graphs \(G\) in \(G(n,1/2)\) are not even in the support of \(G(n,1/2,\omega)\)! Indeed, if we let \(f_G(x)\) be the function that ignores \(x\) and is equal to \(1\) if \(G\) has a clique of size \(\geq 100 \log n\) and to \(0\) otherwise then clearly no pseudo-expectation operator (which satisfies \(\pE 0 = 0\)) can satisfy \eqref{eq:pseudo-calibration} for \(f\). Yet considering this function \(f\) is somewhat “unfair” because a computationally bounded observer will not be able to compute it. Hence we would want to that any pseudoexpectation we construct be pseudocalibrated for every “simple” function — one that captures the kind of reasoning that we expect a computationally bounded Bayesian observer to be capable of. As we will see, once we restrict to a (suitable notion of) simple functions, our pseudodistribution will be well defined even for a random graph and hence will yield estimates forthe probabilities over this hypothetical object (i.e., the \(\omega\)-sized clique) that does not exist.
The “pseudocalibration” condition \eqref{eq:pseudo-calibration} might seem innocent, but it turns out to imply many useful properties. In particular is not hard to see that it implies that for every simple strong constraint of the clique problem - a function \(f\) such that \(f(G,x)=0\) for every \(x\) that is a characteristic vector of an \(\omega\)-clique in \(G\) - it must hold that \(\pE_G f_G = 0\).
For every graph \(G\), let \(p_G\) be a degree \(d/2\) polynomial such that \(p(x)=0\) for every characteristic vector \(x\) of an \(\omega\)-clique in \(G\). Prove that if a map \(G\mapsto\pE_G\) where \(\pE_G\) is a degree \(d\) pseudo-distribution operator is pseudo-calibrated with respect to the function \(f_G(x)=p_G(x)^2\) then it must satisfy that \(\pE_G p_G = 0\) for every graph \(G\).
But even beyond these “hard constraints”, \eqref{eq:pseudo-calibration} implies that the pseudo-expectation satisfies many weak constraints as well, such as the fact that a vertex of high degree is more likely to be in the clique and that if \(i\) is not in the clique then its neighbors are less likely and non-neighbors are more likely to be in it. We will explore these consequences in exercises that follow shortly after describing the pseudodistribution in the next section.
Constructing the Pseudodistribution
As before, we will specify our pseudodistribution by describing the associated pseudoexpectation on a basis of low-degree functions. As in the case of Grigoriev’s theorem, the fact that the pseudo-distribution is over \(\bits^n\) forces \(\pE_G f = \pE_G m(f)\) for any polynomial \(f\) and \(m(f)\) obtained by reducing \(f\) to a multilinear polynomial via the relations \(\{ x_i^2 = x_i\}\). Thus, it will be enough to specify \(\pE x_S\) for every \(|S| \leq d\), \(S \subseteq [n]\) where, as before, \(x_S = \Pi_{i \in S} x_i\).
We will choose our pseudodistribution to be pseudocalibrated for every low-degree function in both the graph \(G\) (seen as a \({n \choose 2}\) indicator variables) and \(x\) with respect to the planted distribution \(G(n,\frac{1}{2},\omega).\)Where does the planted distribution come from? The lower bound result makes no mention of the planted distribution \(G(n,1/2,\omega)\) and only refers to an actual random graph. Thus it might seem strange that we base our pseudo-distribution on the planted distribution via \eqref{eq:pseudo-calibration}. > One way to think about the planted distribution is that it corresponds to a Bayesian prior distribution on the clique. Note that this is the maximum entropy distribution on cliques of size \(\omega\), and so it is a natural choice for a prior per Jaynes’s principle of maximum entropy. Our actual pseudo-distribution can be viewed as correcting this planted distribution to a posterior that respects simple inferences from the observed graph \(G\).
For any polynomial \(p(G,x)\) (identifying \(G\) with its adjacency matrix in \(\bits^{\binom{n}{2}}\)), let \(\deg_G(p)\) and \(\deg_x(p)\) be the degrees of \(p\) in \(G\) and \(x\) variables respectively. Finally, for any polynomial \(p(G,x)\), let \(p(G,x)_{\leq d, \leq \tau}\) be the truncation of \(p\) obtained by dropping the monomials of degree with \(deg_x\) exceeding \(d\) or \(\deg_G\) exceed \(\tau\).
To satisfy the pseudocalibration requirement discussed above, we define the mass function of the pseudodistribution \(\mu(G,x)\) by low-degree truncation of the planted distribution’s mass function \(\mu_{planted}(G,x)\):For every \(G\) and \(x\), \(\mu_{planted}(G,x)\) equals the probability that \((G,x)\) is obtained from \(G(n,1/2,\omega)\). \[ \mu(G,x) = \mu_{planted}(G,x)_{\deg_x \leq d,\deg_G \leq \tau}. \]
In other words, the pseudodistribution is obtained by taking a low-degree (in both \(G\) and \(x\) variables) truncation of the planted probability distribution. As an exercise, please verify that this still satisfies the normalization condition at least in expectation, in the sense that \(\E_{G\sim G(n,\1/2)} \sum_{x\in\bits^n}\mu(G,x) = 1\). It is important to note that most graphs \(G\) from \(G(n, \frac{1}{2})\) are not even in the support of the planted distribution and thus, if we didn’t truncate \(\mu_{planted}(G,x)\), the pseudodistribution will be concentrated on the tiny fraction of the graphs in \(G(n,\frac{1}{2})\) that do have an actual large clique. The truncation above, however, will curb this spiky behavior and at the same time allow the pseudodistribution \(\mu\) to mimic the low-degree behavior of \(\mu_{planted}\) very well.
The above definition indeed gives us the pseudocalibration property that we wanted: \[ \E_{G\in G(n,1/2)} \pE_G f_G = \E_{G\in G(n,1/2,\omega)} f_G \label{eq:sat-calib} \] for every \(f\) with \(\deg_x(f) \leq d\) and \(\deg_G(f) \leq \tau\). Indeed we can write \(\mu_{planted}\) as a polynomial in \(G,x\) and write it as \(\mu_{planted} = \mu + \mu'\) where \(\mu\), as we defined it, corresponds to the monomials in this polynomial of \(x\)-degree at most \(d\) and \(G\)-degree at most \(\tau\). Then the RHS of \eqref{eq:sat-calib} correspnds to \(\iprod{f,\mu} + \iprod{f,\mu'}\) where the inner product sums up over all \(G,x\) and now we only need to use the following exercise:
Let \(f\from\bits^{\ell+m}\to\R\) be a polynomial on \(x\in\bits^\ell,y\in\bits^m\) of \(x\)-degree at most \(d\) and \(y\)-degree at most \(d'\). Prove that for every function \(\chi_S\chi'_T = \prod_{i\in S}(1-2x_i) \prod_{j\in T}(1-2y_j)\), if either \(|S|>d\) or \(|T|>d'\) then \(\sum_{x,y \in \bits^{\ell+m}} f(x,y)\chi_S(x)\chi_T(y) = 0\).
Explicitly calculating the polynomial
To obtain explicit expressions for the pseudoexpectations corresponding to \(\mu\), it is helpful to work in a convenient basis for functions of the graph \(G\). We work with the parity/Fourier basis (this choice is made as we’d like an orthonormal basis w.r.t. the random distribution \(G(n,\frac{1}{2})\)) and derive explicit expressions for the pseudoexpectation values next.
For \(\pE = \pE_\mu\) corresponding to \(\mu\) defined above, we’ll choose \(\tau = \Theta(d/\epsilon^2)\) and \(\omega = \Theta(n^{1/2 - \epsilon})\) and analyze the \(\pE_{\mu}\) operator so obtained.
\(\pE_G[x_S]\) for any \(S \subseteq [n]\) is a function of the graph \(G\) and thus, can be expanded as:
\[ \pE_G[x_S] = \sum_{T \subseteq {n \choose 2}} \widehat{\pE_G[x_S]}(T) \chi_T(G), \] where \(\chi_T(G) = \Pi_{e \in T} (1-2G_e)\) and \(G_e\in \bits\) is the \(e^{th}\) element of \(G\)’s adjacency matrix.
The next exercise asks you to compute the Fourier coefficients of \(\pE[x_S]\) from the definition above.
Prove the following explicit formula for the Fourier coefficients of the pseudoexpectation defined above. \[ \widehat{\pE[x_S]}(T) = \begin{cases} \left(\frac{\omega}{n} \right)^{|{\mathcal{V}}(T) \cup S|} & \quad \text{if $| {\mathcal{V}}(T)| \leq \tau$}\\ 0 & \quad \text{otherwise}\mper \end{cases} \]
The above definition will incur a slight error in satisfing the clique constraints (\(x_ix_j = 0\) if \(G_e=0\)). This is not a big deal - one way to correct this issue is to define a slightly more clever way of low-degree truncation of \(\mu_{planted}\). The next exercise explores this modification.
Let \(\pE\) be obtained by a truncation, the degree of which depends on the monomials involved in the following way: \[ \widehat{\pE[x_S]}(T) = \begin{cases} \left(\frac{\omega}{n} \right)^{|{\mathcal{V}}(T) \cup S|} & \quad \text{if $| {\mathcal{V}}(T) \cup S| \leq \tau$}\\ 0 & \quad \text{otherwise}\mper \end{cases} \] Show that with probability \(1\), if \(S \subseteq [n]\) of size at most \(d\) is not a clique in \(G\), then \(\pE[x_S] = 0\) for the \(\pE\) defined above.
For \(x\), the degree is decided by the degree of our pseudodistribution, which we will denote by \(d\) in the following. Choosing an upper bound \(\tau\) on degree of the function \(f(G,x)\) in the \(G\) variables is more subtle and is decided based on a trade-off between two competing factors: if we choose \(\tau\) to be too small, the pseudodistribution will not satisfy the positive semidefiniteness condition and if we choose it to be too large, we will see that \(\pE[1]\) will have too high a variance around \(1\). We will thus choose \(\tau\) be the “goldilocks” value as we will soon see.
The next exercise verifies that \(\pE\) so defined satisfies the normalization condition.
Show that with high probability, \(\pE[1] = 1 \pm n^{-\Omega(\epsilon)}\) and \(\pE[\sum_{i \in [n]} x_i] = \omega \cdot (1 \pm n^{-\Omega(\epsilon)})\).
We have thus established that 1) \(\pE[1] \approx 1\), 2) \(\pE[\sum_{i \in [n]} x_i] \approx \omega\), and 3) \(\pE[x_S] = 0\) for every \(S \subseteq [n]\) which is not a clique in \(G\).
\(\pE\) is Positive Semidefinite
What remains to argue is that the \(\pE\) defined above satisfies the positivity/positive semidefiniteness property.
With high probability over \(G\) from \(G(n,1/2)\), for \(\tau = \Theta(d/\epsilon^2)\) and \(\omega = \Theta(n^{1/2-\epsilon})\) every polynomial \(p\) of degree at most \(d\) satisfies, \[ \pE_G[p(x)^2] \geq 0 \]
Given the principled way we constructed the pseudoexpectation, one would expect a proof of positive semidefiniteness that relies on certain nice aspects of the planted distribution and how they play with the random distribution. Unfortunately, we do not know of a simple argument along these lines (but is an excellent open question to find one!). The proof we present in the next section is delicate and technical and involves an approximate orthogonalization of the associated moment matrix.
The following exercise shows that at least our pseudo-distribution is positive semidefinte “in expectation” and for polynomials that are obtained as “simple” functions of the graph:
Prove that if \(f\from\bits^{\binom{n}{2}}\times\bits^n\to\R\) has \(G\)-degree \(\leq \tau\) and \(x\)-degree \(\leq d/2\) then \(\E_{G\in {\mathcal{G}}(n,1/2)} \pE_G f_G^2 \geq 0\).
The pseudo-distribution as Bayesian probabilities.
If we truncate to \(G\) degree \(0\) then we completely ignore the graph and
hence we would set the expectation \(\pE x_i\) to be \(\tfrac{\omega}{n}\)
for every \(i\). If we consider functions with \(G\)-degree \(1\) then we
start taking into account the correlations between the degree of the
vertex (which is a linear function in the graph) and the probability
that it is in the clique, and hence slightly update the probabilities to
increase the likelihood of some vertices and decrease the others.
When we consider functions with \(G\)-degree \(2\) then we can also take
into account triangle statistics. Of course if we went all the way to
\(G\)-degree \(3\log n\) then in a planted graph we would be able to
completely identify the vertices of the clique and in a random graph the
pseudo-distribution will stop making sense (as in that \(\pE 1\) will
start having huge variance). Thus these pseudo distributions can be
thought of as gradually reducing our uncertainty as we spend more and
more time on the computation.
References
Alon, Noga, Alexandr Andoni, Tali Kaufman, Kevin Matulef, Ronitt Rubinfeld, and Ning Xie. 2007. “Testing K-Wise and Almost K-Wise Independence.” In STOC, 496–505. ACM.
Alon, Noga, Michael Krivelevich, and Benny Sudakov. 1998. “Finding a Large Hidden Clique in a Random Graph.” In SODA, 594–98. ACM/SIAM.
Applebaum, Benny, Boaz Barak, and Avi Wigderson. 2010. “Public-Key Cryptography from Different Assumptions.” In STOC, 171–80. ACM.
Arora, Sanjeev, Boaz Barak, Markus Brunnermeier, and Rong Ge. 2010. “Computational Complexity and Information Asymmetry in Financial Products (Extended Abstract).” In ICS, 49–65. Tsinghua University Press.
Austrin, Per, Mark Braverman, and Eden Chlamtac. 2013. “Inapproximability of Np-Complete Variants of Nash Equilibrium.” Theory of Computing 9: 117–42.
Barak, Boaz, Samuel B. Hopkins, Jonathan A. Kelner, Pravesh Kothari, Ankur Moitra, and Aaron Potechin. 2016. “A Nearly Tight Sum-of-Squares Lower Bound for the Planted Clique Problem.” IEEE Symposium on Foundations of Computer Science, FOCS.
Berthet, Quentin, and Philippe Rigollet. 2013. “Complexity Theoretic Lower Bounds for Sparse Principal Component Detection.” In COLT, 30:1046–66. JMLR Workshop and Conference Proceedings. JMLR.org.
Bogdanov, Andrej, and Luca Trevisan. 2003. “On Worst-Case to Average-Case Reductions for NP Problems.” In FOCS, 308–17. IEEE Computer Society.
Feige, Uriel, and Robert Krauthgamer. 2003. “The Probable Value of the Lovász–Schrijver Relaxations for Maximum Independent Set.” SIAM J. Comput. 32 (2): 345–70.
Feigenbaum, Joan, and Lance Fortnow. 1991. “On the Random-Self-Reducibility of Complete Sets.” In Structure in Complexity Theory Conference, 124–32. IEEE Computer Society.
Hajek, Bruce E., Yihong Wu, and Jiaming Xu. 2015. “Computational Lower Bounds for Community Detection on Random Graphs.” In COLT, 40:899–928. JMLR Workshop and Conference Proceedings. JMLR.org.
Hazan, Elad, and Robert Krauthgamer. 2009. “How Hard Is It to Approximate the Best Nash Equilibrium?” In SODA, 720–27. SIAM.
Javadi, Hamid Haj Seyed, and Andrea Montanari. 2015. “The Hidden Subgraph Problem.” CoRR abs/1511.05254.
Jerrum, Mark. 1992. “Large Cliques Elude the Metropolis Process.” Random Struct. Algorithms 3 (4): 347–60.
Juels, Ari, and Marcus Peinado. 1998. “Hiding Cliques for Cryptographic Security.” In SODA, 678–84. ACM/SIAM.
Karp, Richard M. 1976. “The Probabilistic Analysis of Some Combinatorial Search Algorithms.” In Algorithms and Complexity (Proc. Sympos., Carnegie-Mellon Univ., Pittsburgh, Pa., 1976), 1–19. Academic Press, New York.
Koiran, Pascal, and Anastasios Zouzias. 2014. “Hidden Cliques and the Certification of the Restricted Isometry Property.” IEEE Trans. Information Theory 60 (8): 4999–5006.
Kucera, Ludek. 1995. “Expected Complexity of Graph Partitioning Problems.” Discrete Applied Mathematics 57 (2-3): 193–212.
Milo, R., S. Shen-Orr, S. Itzkovitz, N. Kashtan, D. Chklovskii, and U. Alon. 2002. “Network Motifs: Simple Building Blocks of Complex Networks.” Science 298 (5594): 824–27. doi:10.1126/science.298.5594.824.
Pevzner, Pavel A, Sing-Hoi Sze, and others. 2000. Combinatorial Approaches to Finding Subtle Signals in Dna Sequences. Vol. 8.